You signed in with another tab or window. Reload to refresh your session.You signed out in another tab or window. Reload to refresh your session.You switched accounts on another tab or window. Reload to refresh your session.Dismiss alert
create table t1(id int primary key, a int, b int, index(a));
create table t2 like t1;
drop procedure idata;
delimiter ;;
create procedure idata()
begin
declare i int;
set i=1;
while(i<=1000)do
insert into t1 values(i, 1001-i, i);
set i=i+1;
end while;
set i=1;
while(i<=1000000)do
insert into t2 values(i, i, i);
set i=i+1;
end while;
end;;
delimiter ;
call idata();
Multi-Range Read 优化
Multi-Range Read(MRR) 优化的主要目的是尽量使用顺序读盘。
select * from t1 where a>=1 and a<=100;
在 InnoDB 中,这个语句会在普通索引 a 上查到主键 id 的值后,再根据一个个主键 id 的值到主键索引上获取整行的数据。而且,在主键索引这棵 B+ 树上,每次只能根据一个主键 id 查到一行数据
这样,随着 a 的值的递增,id 的值就会变成随机的,就会出现随机访问的问题,导致性能降低。
MRR 优化的思路就是,大多数的情况下,数据都是按照主键递增的顺序插入的,所以可以认为如果按照主键递增顺序查询,对磁盘来说就比较接近顺序读,会提高读性能。
MRR 优化后的执行流程:
根据索引 a,定位到满足条件的记录,将 id 值放入 read_rnd_buffer 中
将 read_rnd_buffer 中的 id 进行递增排序
排序后的 id 数组,依次到主键 id 索引中查数据,并作为结果返回
read_rnd_buffer 的大小由 read_rnd_buffer_size 参数控制,如果步骤 1 中 read_rnd_buffer 满了,会先执行步骤 2 和步骤 3,然后清空 read_rnd_buffer,之后再继续执行步骤 1
如果需要稳定的使用 MRR,需要设置 set optimizer_switch="mrr_cost_based=off"(官方文档说法:现在优化器的策略在判断消耗的时候,会更倾向于不使用 MRR,这里把 mrr_cost_based 设置为 off,就是固定使用 MRR)
explain 结果中,如果 Extra 字段中有 Using MRR,就表示用上了 MRR 优化
而且,由于 read_rnd_buffer 中按照主键 id 做了排序,所以最后得到的结果也是按照主键 id 递增的,也就是说如果存在 order by 的话,会用不到 MRR
Batched Key Access
MySQL 5.6 引入了 Batched Key Access (BKA) 算法,是对 NLJ 算法的优化
create temporary table temp_t(id int primary key, a int, b int, index(b))engine=innodb;
insert into temp_t select * from t2 where b>=1 and b<=2000;
select * from t1 join temp_t on (t1.b=temp_t.b);
扩展 hash join
MySQL 不支持哈希 join,但是我们可以在业务端实现类似的流程,实际流程如下:
select * from t1; 取得 t1 的全部 1000 行数据, 在业务端存入一个 hash 结构,比如 c++ 里的 set、PHP 的数组这样的数据结构
select * from t2 where b>= 1 and b <= 2000; 获取 t2 中满足条件的 2000 行数据
join 语句有两种算法,分别是 Index Nested-Loop Join(NLJ) 和 Block Nested-Loop Join(BNL)。对 join 语句的优化,也会涉及到对这两种算法的优化
Multi-Range Read 优化
Multi-Range Read(MRR) 优化的主要目的是尽量使用顺序读盘。
在 InnoDB 中,这个语句会在普通索引 a 上查到主键 id 的值后,再根据一个个主键 id 的值到主键索引上获取整行的数据。而且,在主键索引这棵 B+ 树上,每次只能根据一个主键 id 查到一行数据
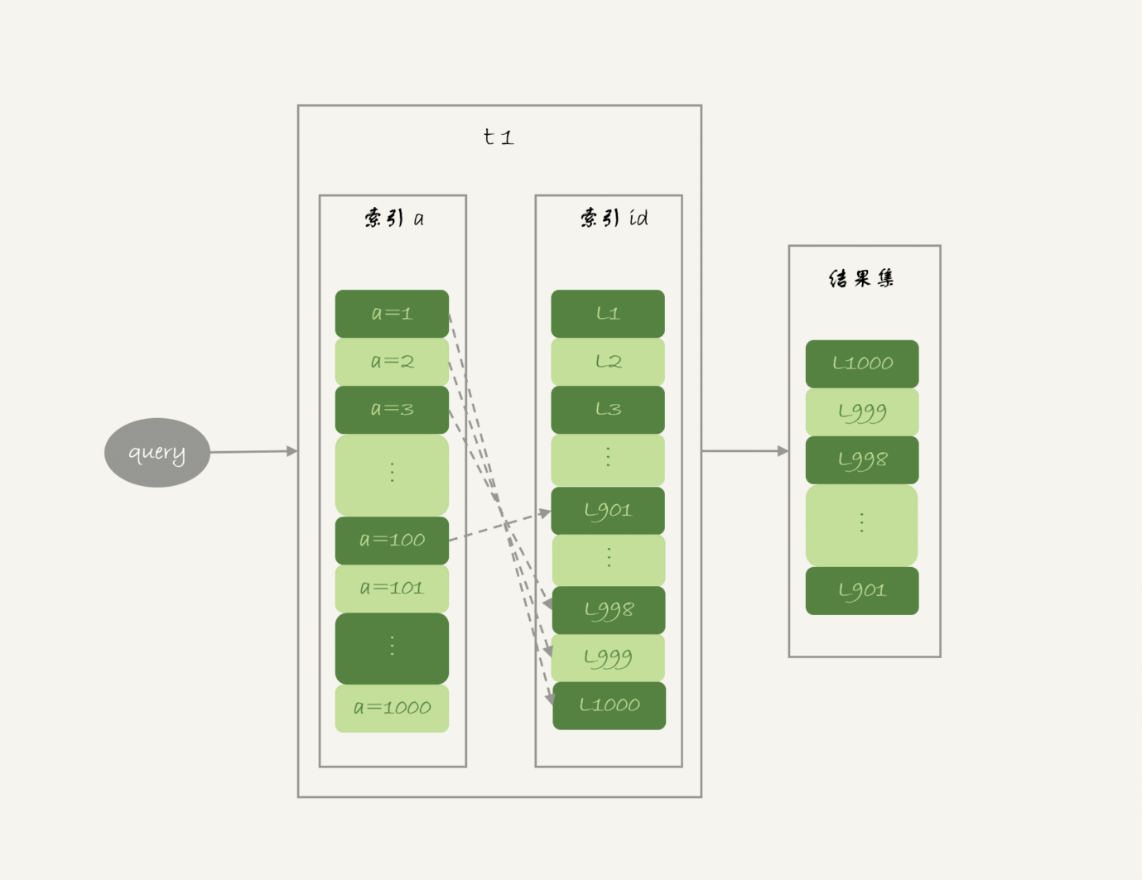
这样,随着 a 的值的递增,id 的值就会变成随机的,就会出现随机访问的问题,导致性能降低。
MRR 优化的思路就是,大多数的情况下,数据都是按照主键递增的顺序插入的,所以可以认为如果按照主键递增顺序查询,对磁盘来说就比较接近顺序读,会提高读性能。
MRR 优化后的执行流程:
read_rnd_buffer 的大小由 read_rnd_buffer_size 参数控制,如果步骤 1 中 read_rnd_buffer 满了,会先执行步骤 2 和步骤 3,然后清空 read_rnd_buffer,之后再继续执行步骤 1
如果需要稳定的使用 MRR,需要设置 set optimizer_switch="mrr_cost_based=off"(官方文档说法:现在优化器的策略在判断消耗的时候,会更倾向于不使用 MRR,这里把 mrr_cost_based 设置为 off,就是固定使用 MRR)
explain 结果中,如果 Extra 字段中有 Using MRR,就表示用上了 MRR 优化

而且,由于 read_rnd_buffer 中按照主键 id 做了排序,所以最后得到的结果也是按照主键 id 递增的,也就是说如果存在 order by 的话,会用不到 MRR
Batched Key Access
MySQL 5.6 引入了 Batched Key Access (BKA) 算法,是对 NLJ 算法的优化
NLJ 算法的执行流程:
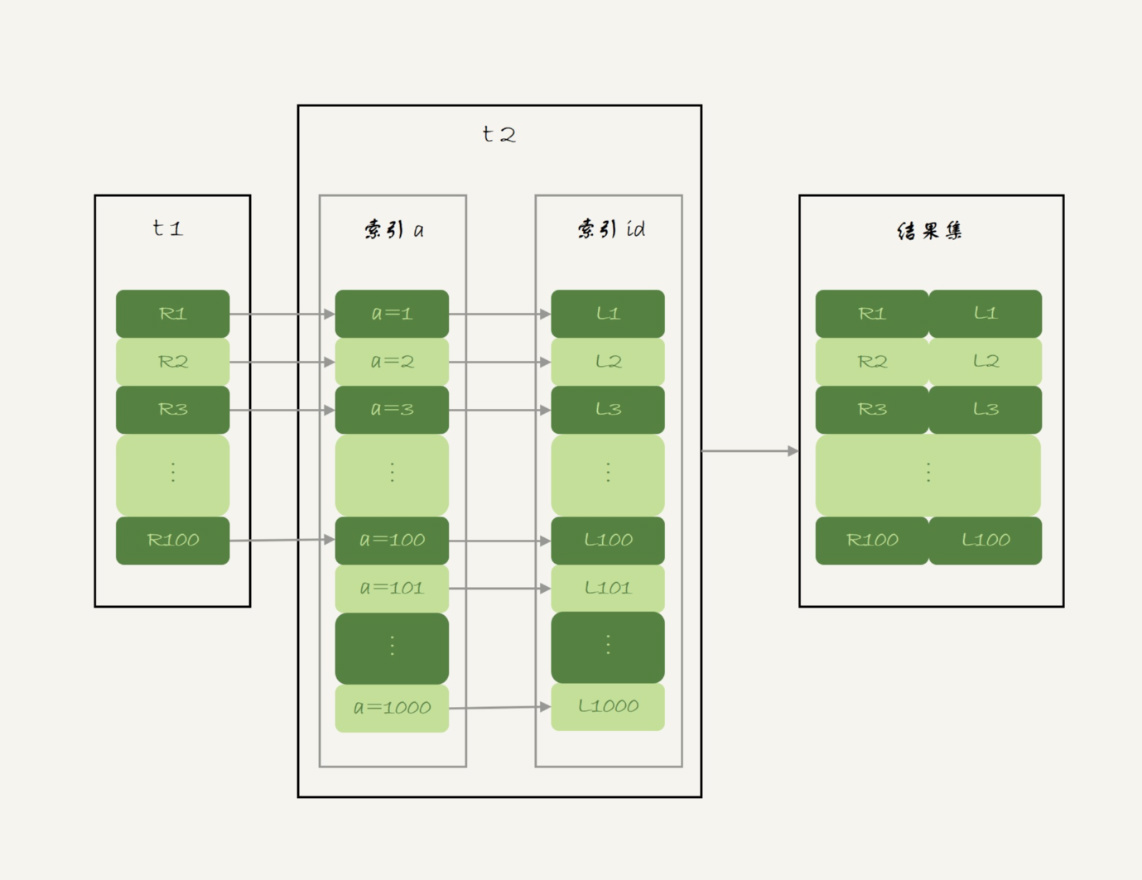
从驱动表 t1,一行行的取出 a 的值,再到被驱动表 t2 去做 join,对于 t2 来说,每次都是匹配一个值
而对于 BKA 算法来说,执行流程就是先把表 t1 的数据取出一部分放到 join_buffer 中,然后在把这部分数据一次性传给 t2,这里就用到了 MRR 的优化
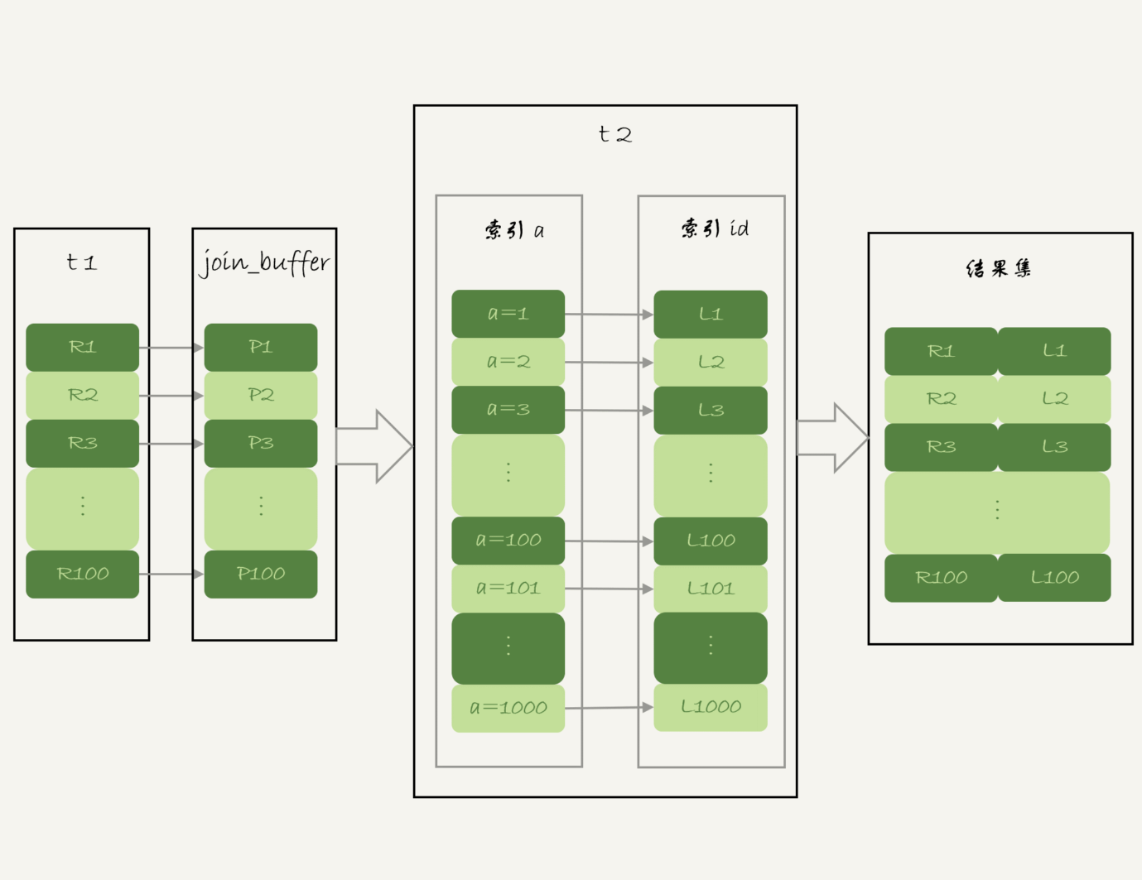
图中,join_buffer 中的 P1 ~ P100 表示的是只会取查询需要的字段
如果要使用 BKA 优化算法,需要先设置:
前两个参数作用是启用 MRR,因为 BKA 算法的优化依赖于 MRR
BNL 算法的性能问题
BNL 算法的优化
通常可以直接在被驱动表上建索引,转成 BKA 算法。
但有些情况下不适合在被驱动表上建索引,就可以使用临时表的方案,大致思路是:
MySQL 不支持哈希 join,但是我们可以在业务端实现类似的流程,实际流程如下:
The text was updated successfully, but these errors were encountered: